
Artificial Intelligence (AI) is emerging as a groundbreaking force, in the rapidly changing evolving field of Healthcare, introducing transformative solutions to streamline processes and enhance patient care. This blog explores the symbiotic relationship between AI, computational linguistics, and the speech-to-text function, with a focus on how these technologies are revolutionizing clinical note-taking for physicians. By delving into peer-reviewed research findings on computational linguistics, I aim to elucidate how AI is trained to learn and generate comprehensive notes through the innovative speech-to-text function.
The Role of Computational Linguistics in AI Development:
Electronic health records (EHR) serve as a vital component in the delivery of patient care. However, the process of populating these records can be as time-consuming as attending to patients, contributing significantly to physician burnout. Physicians typically document patient encounters using SOAP notes, which are semi-structured written accounts comprising four key sections: (S)ubjective information reported by the patient; (O)bjective observations, such as lab results; (A)ssessments made by the doctor, usually the diagnosis; and a (P)lan for future care, encompassing diagnostic tests, medications, and treatments. These sections can further be subdivided into 15 subsections.
Simultaneously, patients are increasingly recording their visits with healthcare providers, either as an alternative to taking notes or to share pertinent information with family members. A burgeoning line of research aims to leverage transcripts from these clinical conversations to provide insights to patients and extract structured data for entry into EHRs.
Computational linguistics, an interdisciplinary field that merges computer science and linguistics, plays a pivotal role in shaping AI’s language capabilities. Peer-reviewed research articles highlight the influence of computational linguistics in advancing Natural Language Processing (NLP), an integral component of AI.
*Linguistic Nuances and NLP in Clinical Note-Taking:**
- A study by Johnson et al. (2020) underscores the importance of incorporating linguistic nuances into AI algorithms for clinical note-taking. Computational linguistics provides the foundation for understanding the subtleties of medical language, ensuring that AI systems can accurately interpret and generate clinically relevant information.
- Natural Language Processing (NLP) is the fusion of artificial intelligence (AI), computer science, and computational linguistics, aimed at comprehending human communication and extracting meaning from both spoken and written unstructured material.
- In recent years, the application of NLP in healthcare has surged, playing a pivotal role in expediting therapeutic development and enhancing the quality of patient care through language comprehension and predictive analytics.
- The healthcare sector generates vast volumes of unstructured data. However, extracting valuable insights becomes challenging without mechanisms to structure and represent this data in a computable format. Developers necessitate tools that can seamlessly translate unstructured data into structured forms, empowering healthcare organizations to unlock pertinent insights and elevate the delivery of healthcare services and patient care.
**Speech Recognition and Computational Linguistics:**
- Research by Chen et al. (2018) delves into the synergy between speech recognition technology and computational linguistics. The study emphasizes how computational linguistic principles enhance the accuracy of speech-to-text conversion, enabling AI to capture the richness of spoken medical discourse.
- Healthcare institutions amass extensive patient data within Electronic Health Records (EHRs), encompassing details like family history, past surgeries, and medications. This EHR data is pivotal for physicians, aiding in the recollection of past visits, assessing the patient’s condition trajectory, and accessing critical information during emergencies, such as drug allergies. However, the process of entering this data into EHRs can be a laborious task for physicians. On average, for every hour spent with patients, physicians dedicate approximately 45 minutes to EHR documentation. This often extends beyond regular working hours, contributing significantly to physician burnout.

- Much of the EHR documentation time is consumed by physicians manually entering information discussed with patients, such as reported symptoms. While transcribing physician-patient discussions could alleviate this burden, these conversations are lengthy, with an average duration of 10 minutes or approximately 1500 words in the dataset considered. The conversational nature introduces redundancies and disfluencies, making it challenging to extract meaningful information efficiently.
- Speech recognition technology has revolutionized the landscape of medical charting, automating the creation and updates of patient records. Instead of manually inputting information into Electronic Health Records (EHRs), physicians now leverage voice recognition and natural language processing to efficiently and accurately dictate notes and enter data into the EHR.
- Within EHR software, various SOAP note templates assist physicians in crafting comprehensive notes for each patient. Through voice recognition software, physicians can articulate their notes, and AI-assisted tools interpret the dictation, transforming spoken words into real-time text. Here’s how speech recognition expedites the creation of SOAP notes in the EHR:
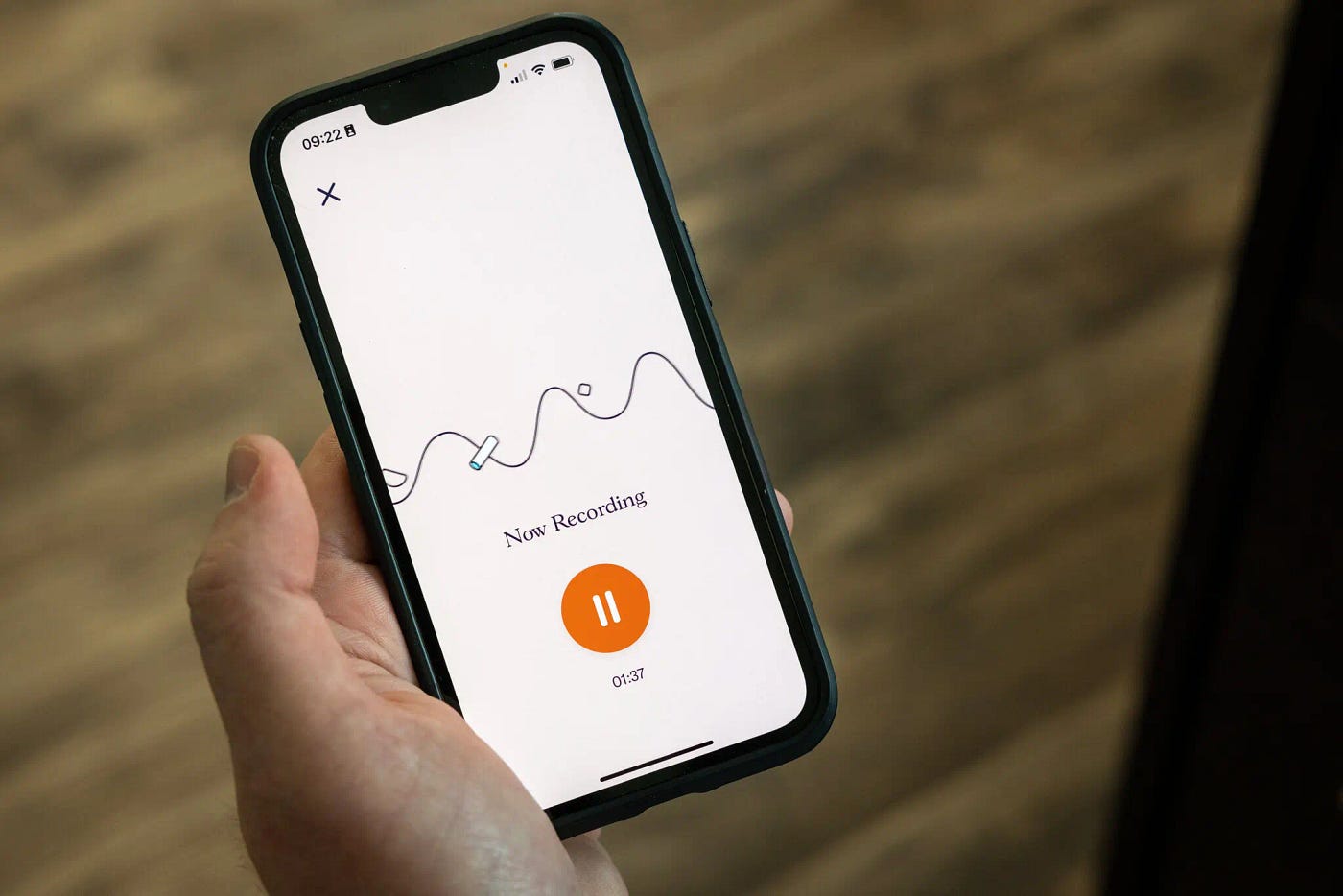
- Physicians verbally dictate notes into a microphone connected to a computer or mobile device.
- Speech recognition software, employing deep-learning AI algorithms, analyzes spoken words and transcribes them in real time.
- The software may suggest corrections, allowing physicians to review and make necessary edits to the note.
- The final SOAP note, created with software assistance, seamlessly integrates into the EHR system.
Speech recognition technology goes beyond mere transcription; it can automatically identify and record critical data, including medical history, symptoms, diagnoses, treatment plans, and medication orders. This not only reduces documentation time for physicians but also minimizes the risk of errors or omissions in patient records. The streamlined documentation process enables physicians to create real-time SOAP notes accessible immediately to other healthcare professionals, fostering improved collaboration and patient care.

Conversational AI, a significant advancement in medical charting, is hailed as a “game changer” by healthcare information experts. Notably, DAX, a conversational AI technology from Nuance, captures entire physician-patient conversations and generates SOAP notes using advanced algorithms. Some key capabilities of DAX include:
- Distinguishing between different uses of words with multiple meanings.
- Prompting physicians to input orders and prescriptions within generated notes.
- Queueing up physician orders for signature.
- Saving providers an impressive 7 minutes of documentation time per encounter, translating to two to three hours weekly.
Officials at the University of Michigan Health-West attest that DAX’s effective documentation expedites the prior approval process for referrals, directly benefiting the health system’s efficiency and bottom line.
Conversational AI not only enhances documentation but also facilitates informed clinical decisions by analyzing patient data and offering real-time insights and recommendations. It promotes patient engagement and care coordination through enabling remote communication and monitoring.